SDXL: A Powerful Latent Diffusion Model for Text-to-Image Synthesis
Posted on 2023/07/02 by GoAPI
Text-to-image synthesis has made significant advancements with the introduction of Stable Diffusion, a latent diffusion model (DM). However, researchers have further improved this state-of-the-art method with the development of SDXL.
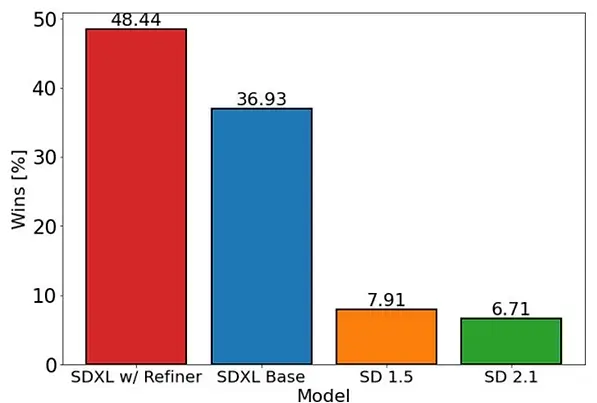
SDXL's Advances
SDXL is a drastically enhanced version of Stable Diffusion, delivering impressive results and outperforming previous versions. The researchers behind SDXL made several design choices, which significantly impacted its performance. These include:
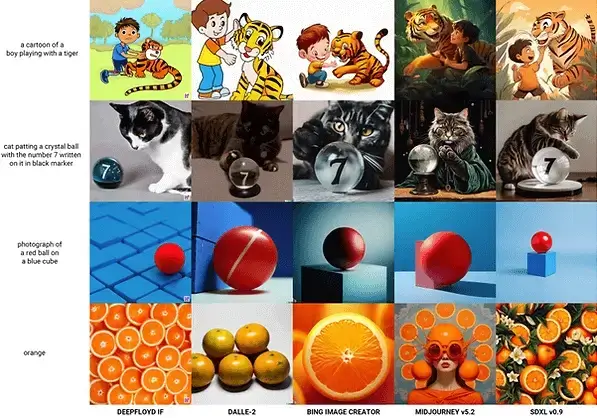
- 1.Larger UNet-backbone: SDXL employs a three times larger UNet backbone compared to its predecessors. This increase in model parameters is mainly due to more attention blocks and a larger cross-attention context. Additionally, SDXL utilizes a second text encoder, enabling improved text-to-image synthesis.
- 2.Novel conditioning schemes: The researchers introduce multiple innovative conditioning techniques that contribute to the improved performance of SDXL. These methods do not require any additional supervision.
- 3.Refinement model: To enhance the visual fidelity of generated samples, SDXL incorporates a refinement model. This model utilizes a post-hoc image-to-image technique, applying a noising-denoising process to the latents produced by SDXL. As a result, the refinement model significantly improves sample quality, especially for detailed backgrounds and human faces.
SDXL's performance is evaluated against previous versions of Stable Diffusion and other black-box state-of-the-art image generators. The comparison demonstrates that SDXL exhibits drastically improved performance and achieves competitive results.
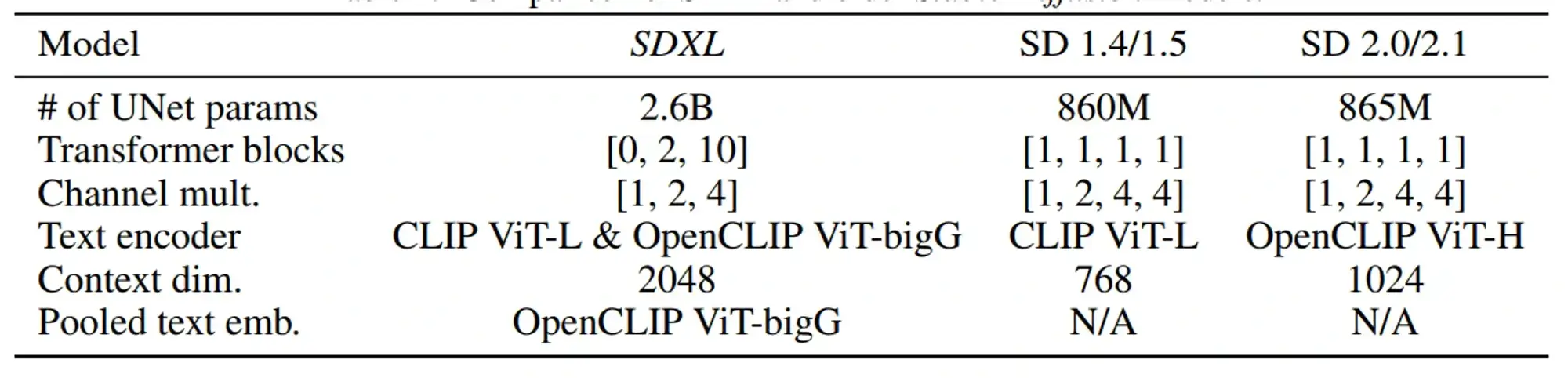
While black-box models are often recognized as state-of-the-art, their opacity restricts the ability to assess and validate their performance accurately. This lack of transparency hampers reproducibility, stifles innovation, and inhibits scientific and artistic progress. Additionally, closed-source strategies make it challenging to assess biases and limitations in an impartial and objective manner.
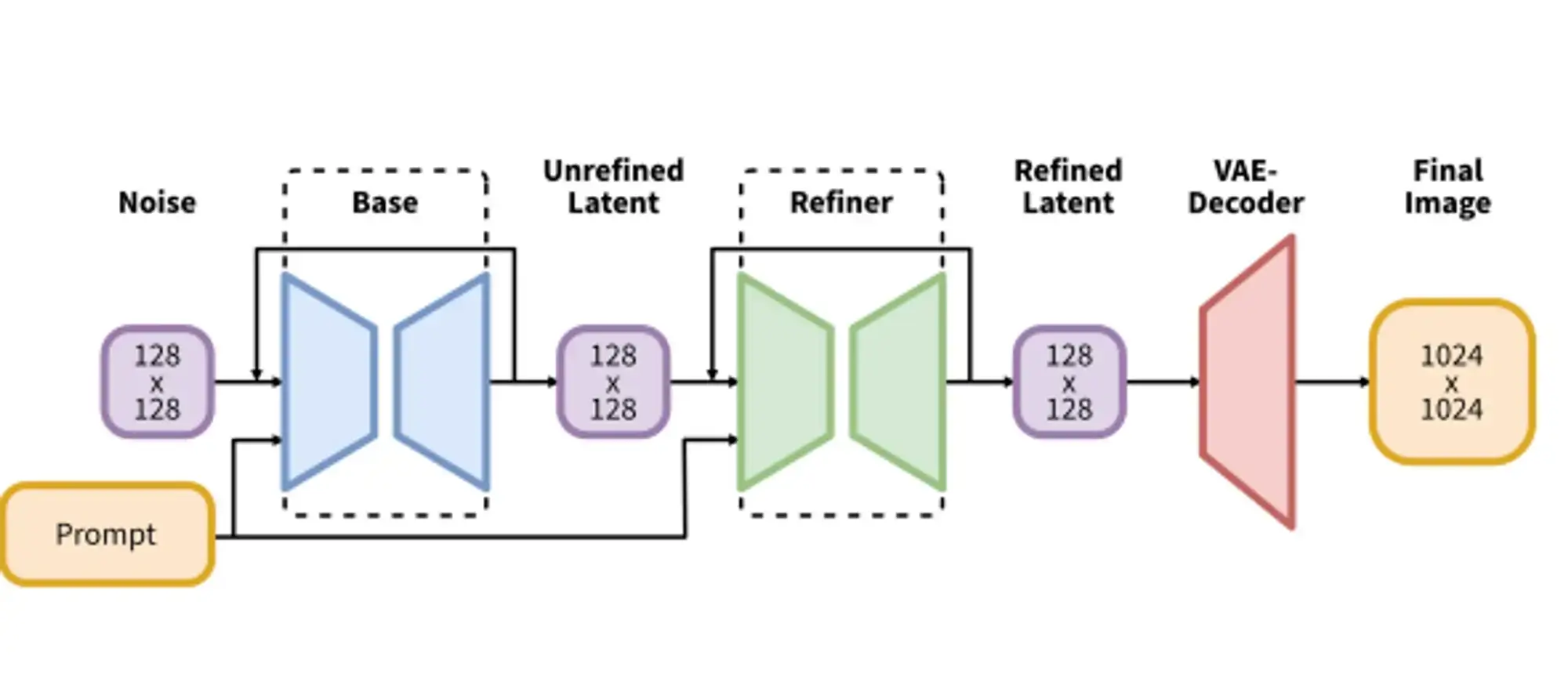
Researchers found that SDXL sometimes generates samples with low local quality. To address this issue, they developed a separate Latent Diffusion Model (LDM). This LDM operates in the same latent space as SDXL but focuses on high-quality, high-resolution data. By applying a noising-denoising process similar to SDEdit on the base model's samples, the refinement model significantly improves the quality of the generated images. It's worth noting that the refinement stage is optional but highly recommended for detailed backgrounds and human faces.
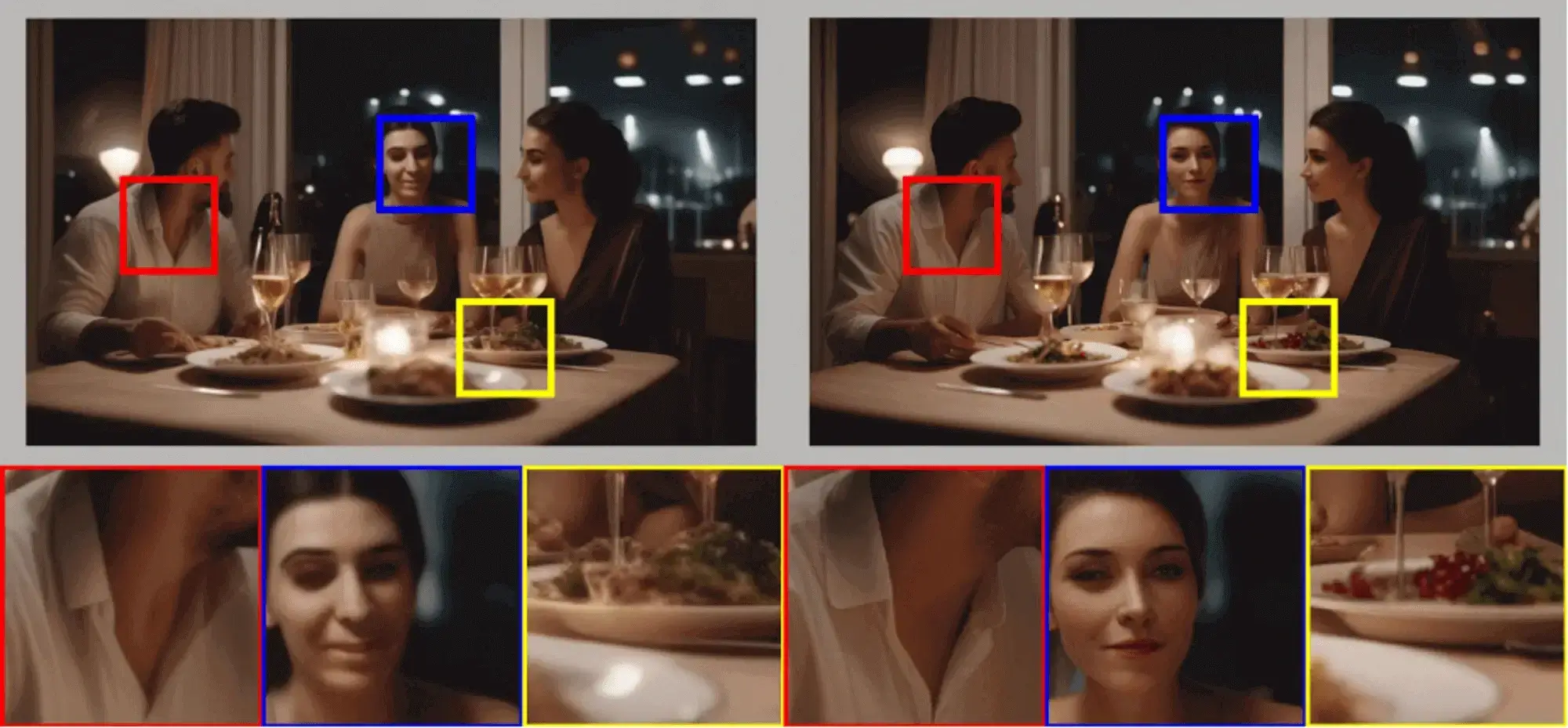
While SDXL demonstrates impressive image synthesis capabilities, there are still areas that could be further improved:
- 1.Single-stage approach: Currently, SDXL utilizes a two-stage approach with an additional refinement model to generate the highest quality samples. However, this approach requires loading two large models into memory, which can hamper accessibility and sampling speed. Future work could focus on developing a single-stage approach that achieves similar performance.
- 2.Text synthesis: Although SDXL exhibits improved text rendering capabilities compared to previous versions of Stable Diffusion, there is room for further enhancement.
- 3.Architecture and distillation: While SDXL shows significant improvements over the original Stable Diffusion, it comes at the cost of increased inference cost (in terms of VRAM and sampling speed). Future research could focus on reducing computational requirements for inference and increasing sampling speed.
Despite its remarkable realism, SDXL does not achieve perfect photorealism. Additionally, the model's training process relies heavily on large-scale datasets, which may introduce unintentional social and racial biases. Addressing biases, refining complex structures, achieving perfect photorealism, improving text rendering, and mitigating concept bleeding are important avenues for future research and optimization.
In conclusion, SDXL represents a significant advancement in text-to-image synthesis. Its design choices, large UNet backbone, novel conditioning schemes, and refinement stage contribute to its outstanding performance. While there are areas for future improvement, SDXL showcases notable strength in image synthesis. Addressing its limitations will pave the way for further research and optimization in this domain.